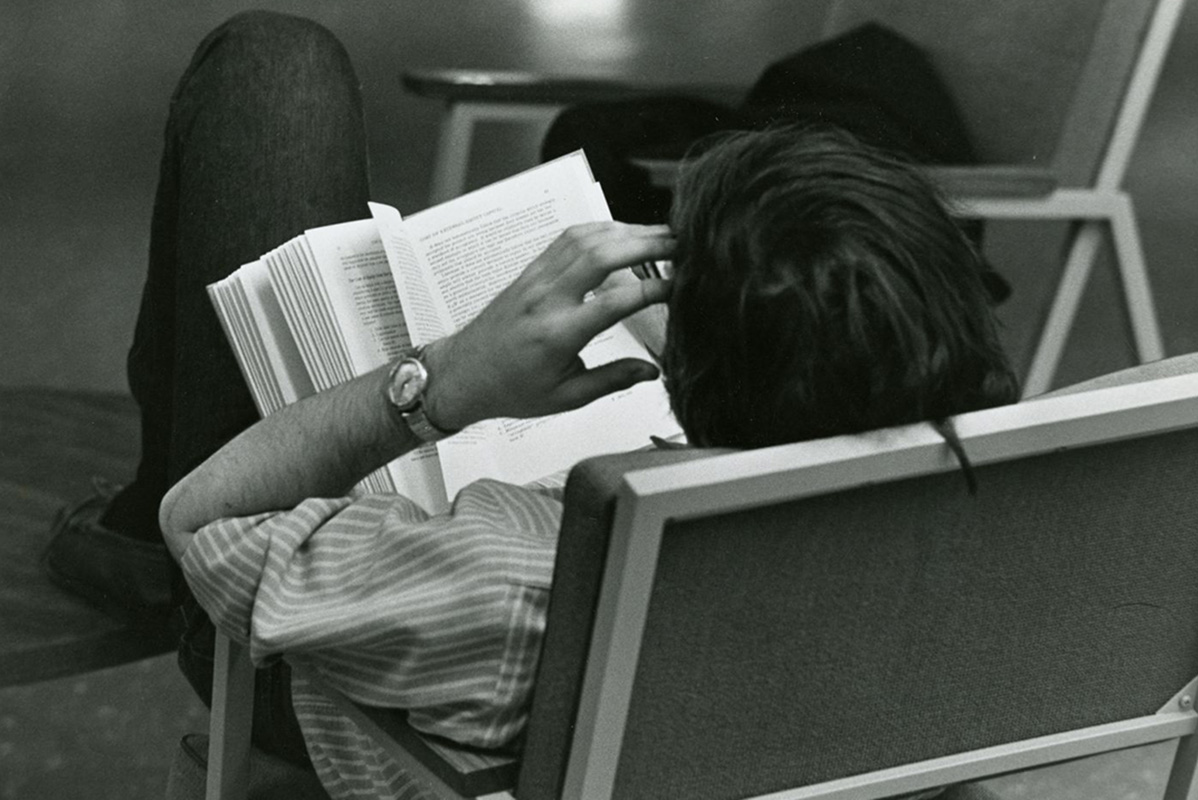
by Lencia Beltran, Open Science Program Coordinator
The introduction of artificial intelligence (AI) and open science in research is reshaping various disciplines and encouraging transparency, collaboration, and innovation.
The first paper examines how Large Language Models (LLMs) can enhance scientific experimentation through improved design, implementation, and data analysis, while proposing a governance framework to ensure responsible use. Another paper details the synergy between open science and AI technologies, emphasizing the potential for personalizing knowledge and augmenting, rather than replacing, human labor. Complementing these discussions, the paper titled "Data Democracy" offers a manifesto on the importance of data ownership and accessibility, advocating for open data and open-source software to democratize AI across diverse fields. Collectively, these selections demonstrate the transformative impact of combining AI with open science principles to create a more equitable and efficient scientific ecosystem.
There are many other readings that explore these and similar topics, and the best part is you can find and access them through the CMU Libraries databases!
Addressing Bias in Big Data and AI for Health Care: A Call for Open Science
Norori, Natalia; Hu, Qiyang; Aellen, Florence Marcelle; Faraci, Francesca Dalia; Tzovara, Athina
Patterns (New York, N.Y.), 2021-10, Vol.2 (10), p.100347-100347, Article 100347
Abstract: Artificial intelligence (AI) has an astonishing potential in assisting clinical decision making and revolutionizing the field of health care. A major open challenge that AI will need to address before its integration in the clinical routine is that of algorithmic bias. Most AI algorithms need big datasets to learn from, but several groups of the human population have a long history of being absent or misrepresented in existing biomedical datasets. If the training data is misrepresentative of the population variability, AI is prone to reinforcing bias, which can lead to fatal outcomes, misdiagnoses, and lack of generalization. Here, we describe the challenges in rendering AI algorithms fairer, and we propose concrete steps for addressing bias using tools from the field of open science. Bias in the medical field can be dissected along three directions: data-driven, algorithmic, and human. Bias in AI algorithms for health care can have catastrophic consequences by propagating deeply rooted societal biases. This can result in misdiagnosing certain patient groups, like gender and ethnic minorities, that have a history of being underrepresented in existing datasets, further amplifying inequalities. Open science practices can assist in moving toward fairness in AI for health care. These include (1) participant-centered development of AI algorithms and participatory science; (2) responsible data sharing and inclusive data standards to support interoperability; and (3) code sharing, including sharing of AI algorithms that can synthesize underrepresented data to address bias. Future research needs to focus on developing standards for AI in health care that enable transparency and data sharing, while at the same time preserving patients’ privacy. Artificial intelligence (AI) has an astonishing potential in revolutionizing health care. A major challenge is that of algorithmic bias. Most AI algorithms need big datasets to learn from, but several groups of the human population are absent or misrepresented in existing datasets. AI is thus prone to reinforcing bias, which can lead to fatal outcomes and misdiagnoses. Here, we describe challenges in rendering AI algorithms fairer, and we propose concrete steps for addressing bias using open science tools.
Request this Title
Open Data and Algorithms for Open Science in AI-driven Molecular Informatics
Brinkhaus, Henning Otto; Rajan, Kohulan; Schaub, Jonas; Zielesny, Achim; Steinbeck, Christoph
Current Opinion in Structural Biology, 2023-04, Vol.79, p.102542-102542, Article 102542
Abstract: Recent years have seen a sharp increase in the development of deep learning and artificial intelligence-based molecular informatics. There has been a growing interest in applying deep learning to several subfields, including the digital transformation of synthetic chemistry, extraction of chemical information from the scientific literature, and AI in natural product-based drug discovery. The application of AI to molecular informatics is still constrained by the fact that most of the data used for training and testing deep learning models are not available as FAIR and open data. As open science practices continue to grow in popularity, initiatives which support FAIR and open data as well as open-source software have emerged. It is becoming increasingly important for researchers in the field of molecular informatics to embrace open science and to submit data and software in open repositories. With the advent of open-source deep learning frameworks and cloud computing platforms, academic researchers are now able to deploy and test their own deep learning models with ease. With the development of new and faster hardware for deep learning and the increasing number of initiatives towards digital research data management infrastructures, as well as a culture promoting open data, open source, and open science, AI-driven molecular informatics will continue to grow. This review examines the current state of open data and open algorithms in molecular informatics, as well as ways in which they could be improved in future.
Request this Title
Generation Next: Experimentation with AI
Charness, Gary; Jabarian, Brian; List, John
National Bureau of Economic Research, 2023, NBER working paper series no. w31679
Abstract: We investigate the potential for Large Language Models (LLMs) to enhance scientific practice within experimentation by identifying key areas, directions, and implications. First, we discuss how these models can improve experimental design, including improving the elicitation wording, coding experiments, and producing documentation. Second, we delve into the use of LLMs in experiment implementation, with an emphasis on bolstering causal inference through creating consistent experiences, improving instruction comprehension, and real-time monitoring of participant engagement. Third, we underscore the role of LLMs in analyzing experimental data, encompassing tasks like pre-processing, data cleaning, and assisting reviewers and replicators in examining studies. Each of these tasks improves the probability of reporting accurate findings. Lastly, we suggest a scientific governance framework that mitigates the potential risks of using LLMs in experimental research while amplifying their advantages. This could pave the way for open science opportunities and foster a culture of policy and industry experimentation at scale.
Request this Title
Human Dimension of Open Science and the Challenges of AI Technologies
Zinchenko, Viktor; Mielkov, Yurii; Polishchuk, Oleksandr; Derevinskyi, Vasyl; Trynyak, Maya; Iehupov, Mykola; Salnikova, Natalia; Nazarov, D.; Juraeva, A.
E3S web of conferences, 2024-01, Vol.474, p.2008
Abstract: Open Science as a major enterprise to enable a citizen science and AI technologies that can provide for the vast amounts of information to be digested by each human persons are argued to be connected to each other by revealing the possibility of the personalization of knowledge and the human dimension of science. The development of the IT sphere is shown to be the history of its personalization, which presents the challenges for handling the present-day AI technologies so that they would augment human labourers, and not replace them.
Request this Title
Data Democracy: At the Nexus of Artificial Intelligence, Software Development, and Knowledge Engineering
Batarseh, Feras; Yang, Ruixin
Elsevier Science, 2020, 266 pages
Abstract: "Data Democracy: At the Nexus of Artificial Intelligence, Software Development, and Knowledge Engineering" provides a manifesto to data democracy. After reading the chapters of this book, you are informed and suitably warned! You are already part of the data republic, and you (and all of us) need to ensure that our data fall in the right hands. Everything you click, buy, swipe, try, sell, drive, or fly is a data point. But who owns the data? At this point, not you! You do not even have access to most of it. The next best empire of our planet is one who owns and controls the world's best dataset. If you consume or create data, if you are a citizen of the data republic (willingly or grudgingly), and if you are interested in making a decision or finding the truth through data-driven analysis, this book is for you. A group of experts, academics, data science researchers, and industry practitioners gathered to write this manifesto about data democracy.
Request this Title
Feature image: "A college student sits back in a chair and reads a book." (c.1985) Found in the Carnegie Mellon University Archives, available online via our CMU Digital Collections.